Data Engineering at Rittman Mead
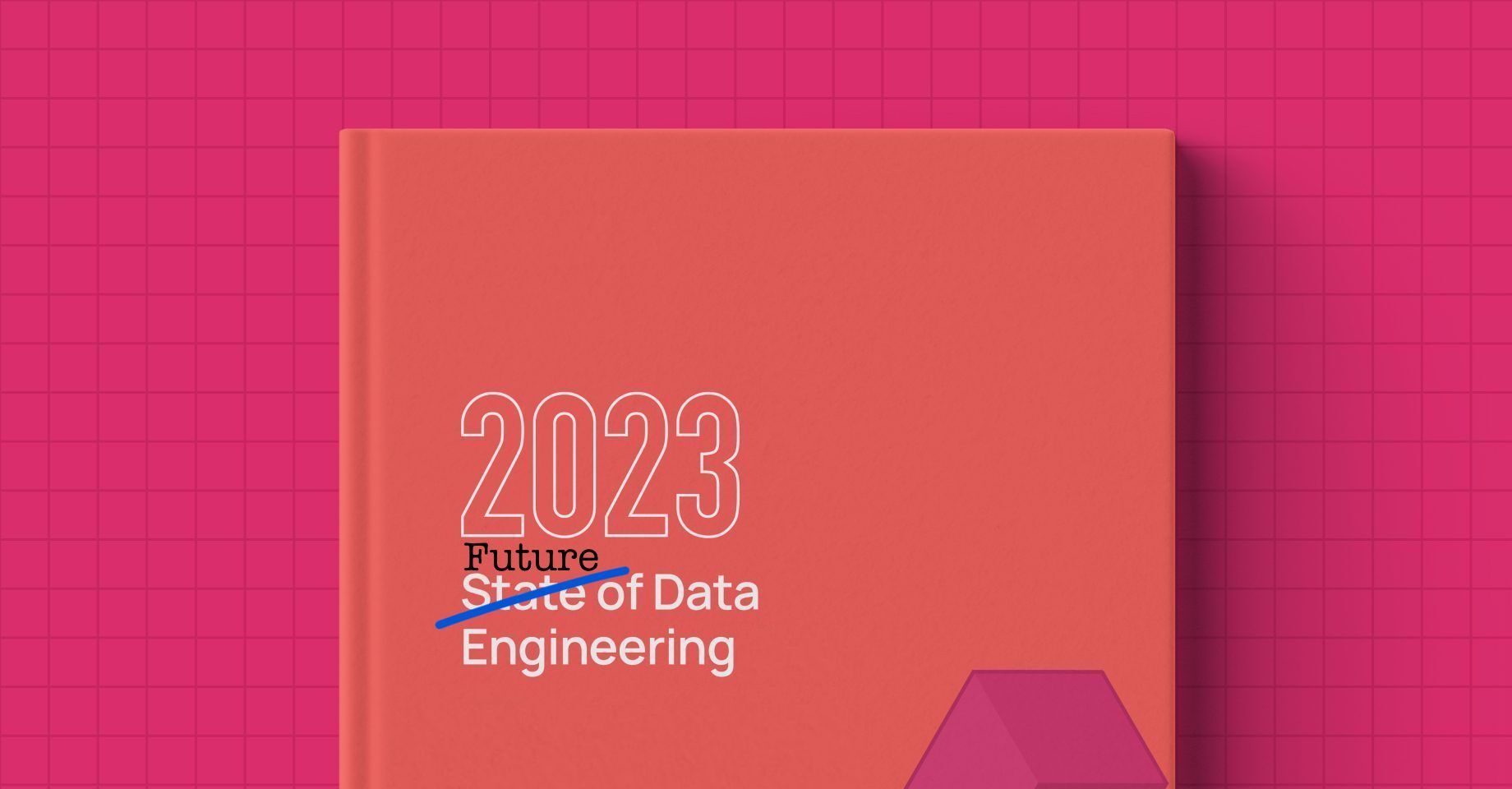
The art of data engineering has been long practiced here at Rittman Mead. With the growing need for data pipelines that transform and deliver data in fast and correct format we decided at the beginning of 2023 to unite a team to work full time on projects or support specific tasks. This team is composed of highly qualified software engineers with a deep knowledge on data pipelines and excellent capabilities of learning new tools.
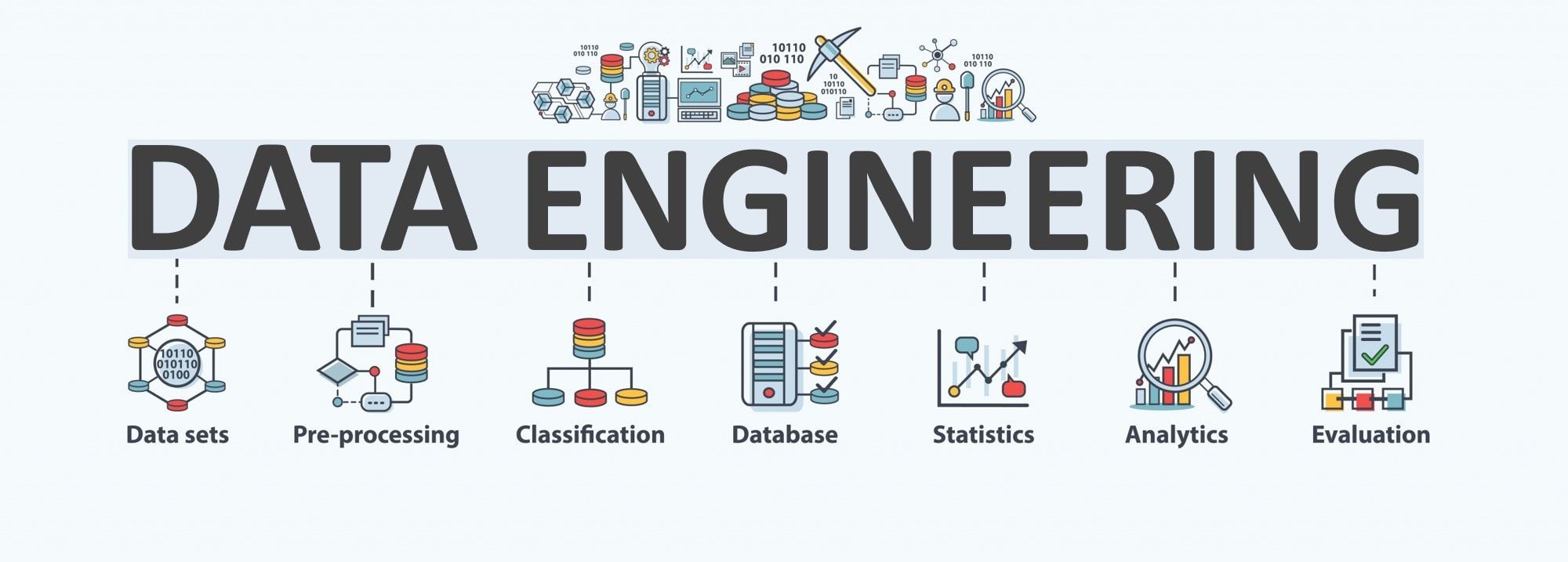
Data Engineering Services at Rittman Mead
We take a holistic approach to data engineering. We see it as a collaborative effort between data engineers, data architects and analysts. This ensures that the data pipelines are designed with the end-users in mind and are optimised for their specific business needs. At its core, it involves building and maintaining the infrastructure that supports data processing and analytics. This includes everything from designing and implementing data storage solutions to developing ETL (extract, transform, load) pipelines or steaming applications that enable data to be moved from one system to another.
One of the key technologies we use for data collection and processing is Oracle Data Integrator (ODI). ODI is a powerful ETL (or ELT) tool that can handle complex data integration scenarios. It allows us to transform large volumes of data into the required format, and load it into a data warehouse or other data storage systems. With the rising need of scalability and quick response times, we also provide streaming solutions that can either work independently or integrated with more common ETL and Data Storage systems.
Alongside our experience in existing streaming tools, such as Apache Kafka or Apache Spark, we have been developing initiatives to mature our knowledge on the recently released product by Oracle for its Cloud Infrastructure (OCI): Oracle Cloud Infrastructure Queue. This will sit perfectly along with the current OCI Data Lake technologies like OCI Object Storage or OCI Data Catalog to make the data processing stage more efficient, scalable and resilient. We are looking forward to sharing more about this with you in the coming weeks.
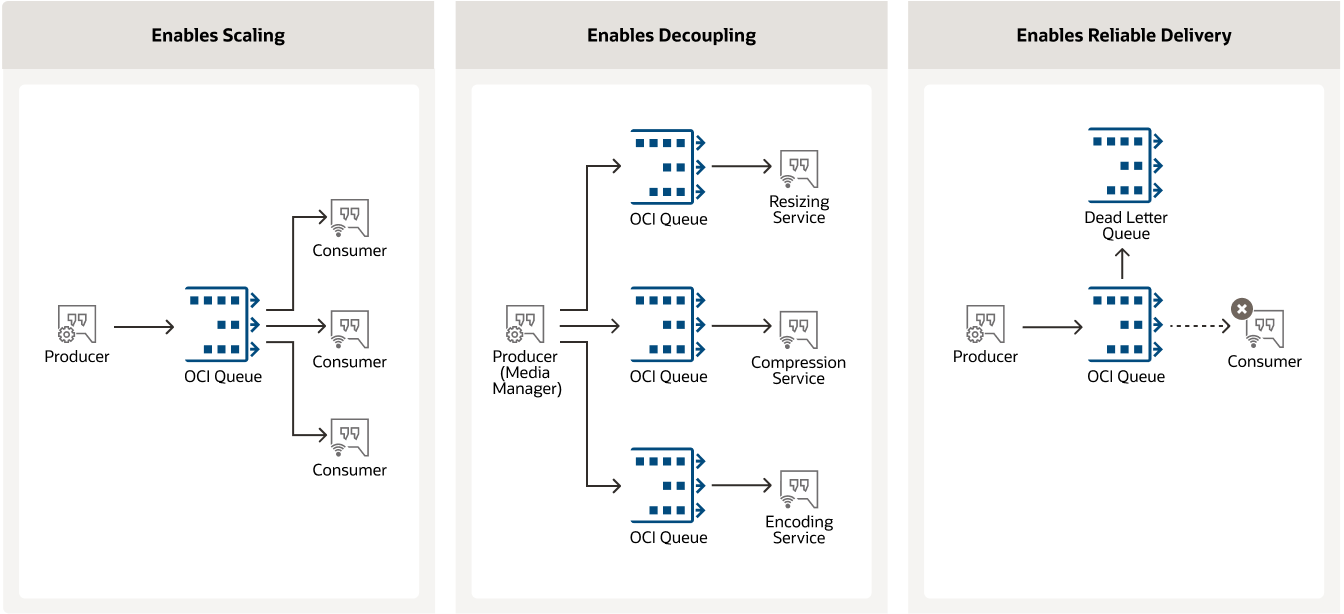
The Future of Data Engineering
A few years ago the concept of data engineering was almost nonexistent. Most companies relied on their on-premises infrastructure to run their daily operations and it was not uncommon to see small teams running data operations activities using a monolithic approach. Times change and with the rise of cloud providers offering robust cloud solutions, companies are now directing a considerable part of their IT on-premises budget to cloud migration projects.
With cloud providers offering a variety of tools making data pipelines seamless, we are now looking to the future of data engineering. We believe there are several trends that are likely to shape the field in the years ahead.
Foundation Teams
We believe data engineers will stop working on a particular product, and instead join newly created foundation teams.
Cloud-Based Data Engineering
Cloud-based platforms offering scalability, flexibility, and cost-effectiveness, will make it easier for businesses to manage and process large volumes of data. As more businesses move to the cloud, cloud-based data engineering will also become increasingly popular. Cloud-based platforms providing a range of advanced tools and services, such as machine learning and artificial intelligence, will help businesses extract valuable insights from their data.
DataOps
DataOps is a new approach to data management that focuses on collaboration and automation. It brings data engineers, data scientists, and other stakeholders together to work on data-related projects, with the goal of improving efficiency and reducing errors. DataOps emphasises automation, using tools and technologies to streamline the data management process reducing the risk of human error.
Data Privacy and Security
As businesses collect and process more data, there is a growing concern about data privacy and security. In response, data engineers will become increasingly focused on building a secure and privacy-focused data infrastructure. This will involve implementing strong security measures, such as encryption and access controls, as well as complying with data protection regulations, such as the General Data Protection Regulation.
Real-Time Data Processing
Businesses will look to process data in real-time, enabling them to make faster and more informed decisions. Real-time data processing involves capturing, processing, and analysing data as it's generated, rather than storing it for batch processing later on. This will require specialised tools and infrastructure, such as real-time data processing engines and stream processing frameworks.
Data Governance and Compliance
As businesses reap the benefits and become more reliant on data, there will be a growing need for effective data governance and compliance. This will involve establishing policies and procedures for data management, ensuring data quality, and complying with the relevant regulations and standards. Data engineers will play a key role in establishing and maintaining these policies and procedures, and in ensuring that data is used in an ethical and responsible way.
Artificial Intelligence and Machine Learning
The topic of the moment and not much to say here. We all know what this is about!