Generative AI: The do-it-all Friend
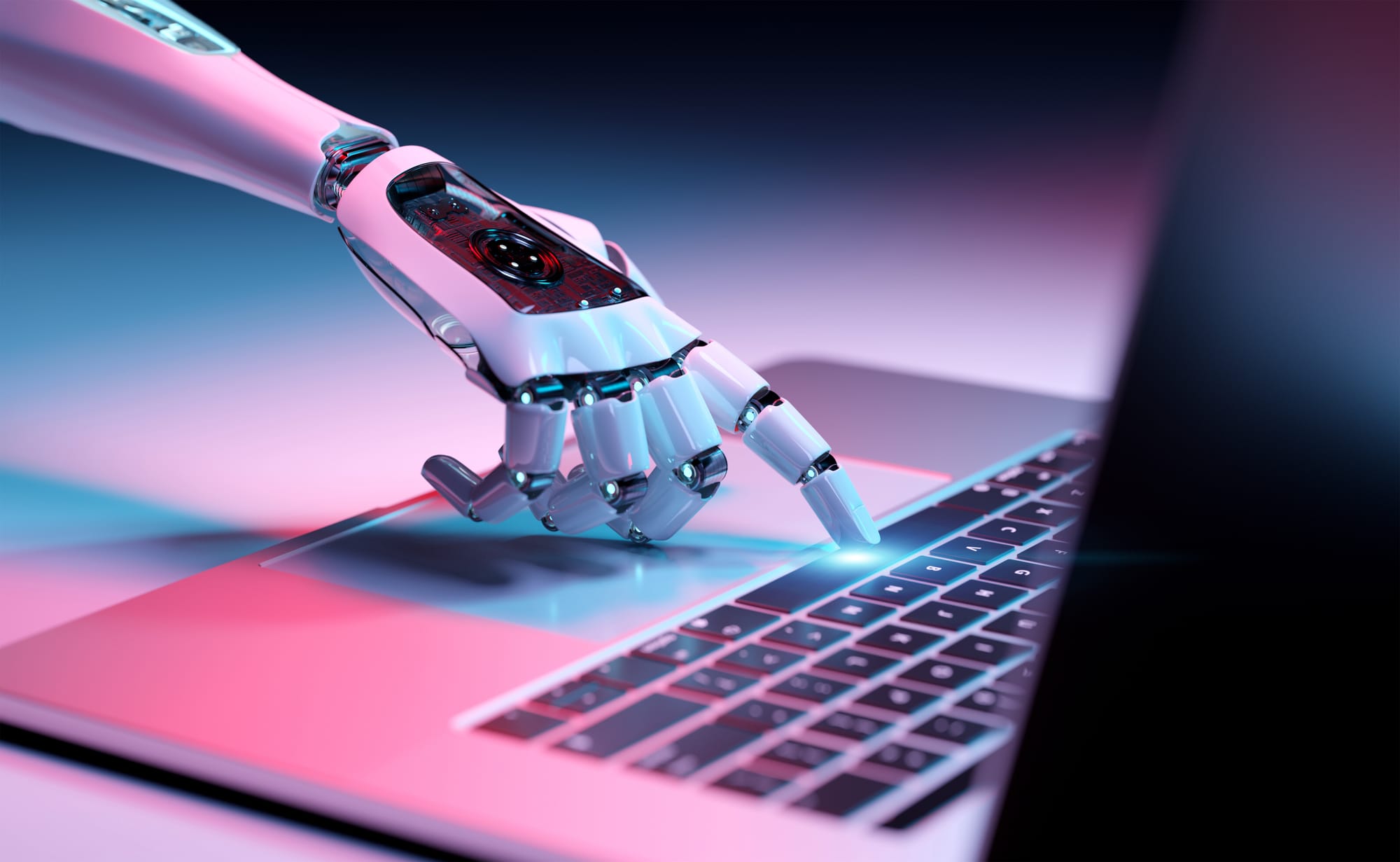
This revolutionary technology, a form of artificial intelligence, can create a whole range of diverse content like text, images, audio, and synthetic data. Although AI's roots trace back to the 1956 Dartmouth Summer Research Project, it was the introduction of generative adversarial networks (GANs) in 2014 that enabled AI to create remarkably authentic images, videos, and audio of real people.
Applications range from improved movie dubbing and educational content to concerns like deepfakes and cybersecurity threats. Large language models with billions or trillions of parameters have ushered in a new era, enabling the creation of engaging text, photorealistic images, and even sitcoms. Despite early challenges with accuracy and bias, the potential of generative AI promises transformative impacts across various sectors, from coding and drug development to product design and supply chain transformation.
Generative AI begins with a prompt, which could be in the form of text, an image, a video, or any input the AI system can handle. Diverse AI algorithms then generate new content in response to the prompt, ranging from essays and problem solutions to realistic fakes created from pictures or audio recordings of a person.
In its early stages, using generative AI involved submitting data through an API or a complex process, requiring developers to work with specialized tools and languages like Python. However, advancements in generative AI now prioritize user-friendly experiences. Pioneers in the field are creating interfaces that allow users to describe requests in plain language. Additionally, users can refine the generated content by providing feedback on style, tone, and other elements after the initial response.
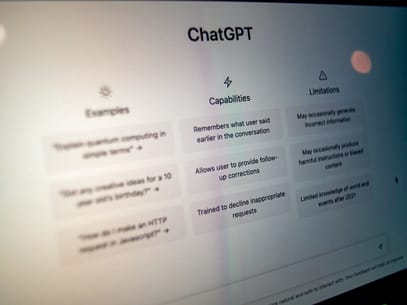
Generative AI works by learning patterns and structures within vast amounts of existing data and then uses those patterns to generate new and original content. How this is achieved can vary depending on the type of generative model being used, but the general steps are as follows:
Data Preparation: The first step is to prepare the source data used to train the AI model which can be anything from text, images, audio, video etc. After collation, some processing is required to clean, normalise and transform the data into a format that is easier to consume by the model. Typically the data is converted into numerical representations such as vectors and matrices, e.g. images are often represented as matrices of pixel values, while text is represented as sequences of word or character vectors.
Model Training: The AI model is then trained on the processed data which involves feeding the data into the model and allowing it to learn the underlying patterns and structures. The choice of model architecture plays a significant role in the learning process. Generative AI models often utilize neural networks, which are complex computational structures inspired by the human brain. Different neural network architectures, such as generative adversarial networks (GANs) and variational auto-encoders (VAEs), have different strengths and weaknesses, and the optimal architecture depends on the specific task and data at hand.
During the training process corrections must be applied to guide the model behaviour and tune the outputs. A method used to aid this corrective process is known as a loss function, which is a mathematical formula that measures the discrepancy between the model's outputs and the desired outputs. The loss function serves as a guide for the model's training, as the parameters used by the model are adjusted to minimise the loss. Some loss functions include the reconstruction loss, which measures the similarity between the model's outputs and the original data, and the adversarial loss, which is used in GANs to encourage the model to generate realistic outputs.
Optimization algorithms are subsequently employed to iteratively update the model's parameters to minimize the loss function. This iterative process involves generating parameters, creating an output based on those parameters, calculating the gradient of the loss function, updating the parameters based on the gradient calculation, and repeating the process iterated.
Once the model has been trained, its performance is evaluated using various metrics that assess the quality of the generated outputs, their similarity to the original data, and their diversity. Some common evaluation metrics for generative AI include the Fréchet inception distance (FID), the Inception score, and the BLEU score.
The entire training process is orchestrated by a set of parameters known as hyperparameters. These parameters, including the learning rate, the number of training epochs, and the regularization strength, control the model's learning journey. They ensure that the model efficiently navigates the landscape of knowledge, avoids overfitting, and ultimately achieves optimal performance.
Regularisation techniques, such as dropout, L1 and L2 regularization, and early stopping, are employed to prevent the model from overfitting to the training data and generalize well to unseen data. These techniques introduce penalties or constraints that discourage the model from becoming overly complex and memorizing the training data instead of learning the underlying patterns.
Data augmentation is another technique used to increase the size and diversity of the training data, thereby reducing the risk of overfitting and improving the model's generalization ability. This technique involves applying various transformations to the existing data, such as flipping images, shifting text, or adding noise, to create new and diverse training examples.
Generation: Once the model is trained, it can be used to generate new content. This is done by providing the model with a prompt or seed, which is a piece of information that helps the model to generate appropriate content. The model then uses its knowledge of the training data to generate new examples that are consistent with the prompt or seed.
Generative AI models use diverse AI algorithms to process and represent content. For instance, in generating text, natural language processing techniques transform characters into sentences, parts of speech, entities, and actions, represented as vectors through various encoding methods. Similar transformations occur for images, converting visual elements into vectors. It's essential to note that these techniques may encode biases, racism, and other traits present in the training data.
Once developers decide on a representation method, they employ specific neural networks to create new content in response to a query or prompt. Techniques like GANs and variational autoencoders (VAEs), which include both a decoder and encoder, are adept at generating realistic human faces, synthetic data for AI training, or imitations of specific individuals.
Recent advancements in transformers like Google's BERT, OpenAI's GPT, and Google AlphaFold have extended the capabilities of neural networks. These models can encode and generate new content across language, images, even proteins, and beyond.
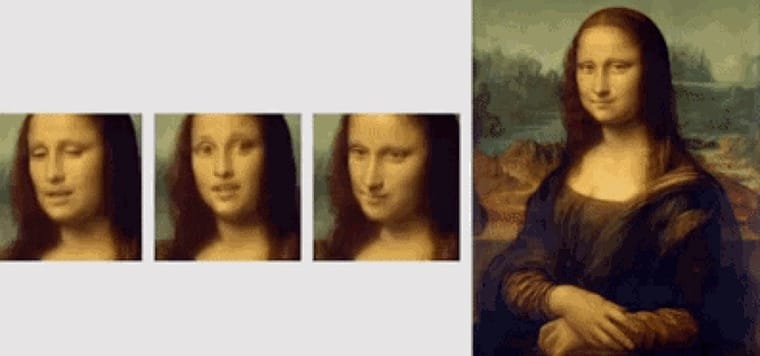
ChatGPT, Dall-E, and Bard – are the distinguished figures in the world of artificial intelligence!
Dall-E: The virtuoso of AI, meticulously trained on an extensive dataset of images paired with nuanced text descriptions. Serving as an exemplar of multimodal AI, Dall-E adeptly navigates connections across various media types, seamlessly merging vision, text, and audio. Its genesis can be traced back to OpenAI's GPT implementation in 2021, and with the advent of Dall-E 2 in 2022, users gained the ability to generate imagery in diverse styles based on their prompts.
ChatGPT: In the AI crescendo of November 2022, ChatGPT emerged as the virtuoso orchestrator. Born from OpenAI's GPT-3.5 implementation, this AI-powered chatbot transcends the ordinary. Departing from the enigmatic API-centric approach, ChatGPT allows users to fine-tune text responses interactively. GPT-4 took center stage on March 14, 2023, enhancing ChatGPT's prowess. Noteworthy is its incorporation of conversation history, simulating authentic dialogues. Microsoft's substantial investment in OpenAI and the integration of GPT into Bing underscored ChatGPT's monumental impact.
Bard: Google's foray into transformer AI techniques resulted in Bard, an early pioneer. Although initially encountering turbulence, Bard underwent a transformation. Hastened to the public sphere following an astronomical misunderstanding, it was crafted on a streamlined version of Google's LaMDA language models. Despite initial hiccups experienced by Microsoft and ChatGPT, Google revealed an evolved Bard, powered by the advanced LLM, PaLM 2. This iteration enables Bard to respond with efficiency and visual finesse to user queries.
Unsurprisingly, the introduction and wide use of such a powerful tools has stirred business disruptions, fuelling predictions of significant workforce changes, including potential job cuts and extensive re-skilling efforts. It's worth noting that, at present, some caution is still necessary as the outputs generated by AI may not always be entirely accurate.
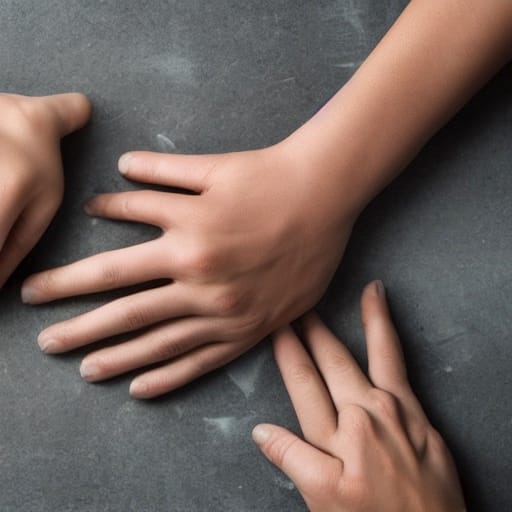